An experimental design to investigate the effects of several explanatory variables, in this context called factors, on a single response variable. Each factor takes only a small number of different values (typically two), which may not be quantitative and are called levels. For example, in an agricultural context the two levels might be two different varieties of a plant. Sir Ronald Fisher introduced the term ‘factor’ in 1929 and used the description ‘factorial design’ as a chapter heading in his seminal work The Design of Experiments, published in 1935.
An experiment with k two-level factors (the upper and lower levels) is called a 2k-factorial. The standard notation denotes factors by capital letters A, B,⋯. Each experimental unit is subject to one or other of the levels of each factor. Lower-case letters are used to indicate factor combinations, so ac indicates that A and C occur at the higher level for that experimental unit, and other factors occur at the lower level. The combination with all factors at the lower level is indicated as (1), so the eight treatment combinations for a 23-factorial are
A 23-factorial enables one to estimate the three main effects (see below) as well as the three interactions involving two variables and the three-variable interaction. The main effect of A compares the mean value of the observations at the higher level (a, ab, ac, abc), for example, with those at the lower level ((1), b, c, bc).
When 2k is large it may not be feasible to maintain constant testing conditions. One solution is to test fractions at a time using randomized blocks:
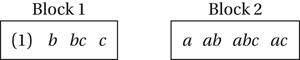
Suppose that the values in the second block of experiments are much larger than those in the first block. It is impossible to tell whether this is because of a difference between the two blocks or a difference between the two levels of A: the effects of A and of the blocks are said to be confounded. A better treatment allocation, in which it is the three-variable interaction that is confounded, is
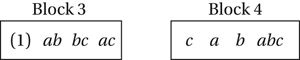
It will be seen that each of the treatment combinations in block 3 has an even number of letters in common with abc while each of those in block 4 has an odd number of letters in common with abc. In the previous example the reference combination was a. The reference combination, which determines the composition of the blocks, is called the defining contrast. An experiment using all four of these blocks provides an example of partial confounding, since two quantities of interest (the main effect of A and the ABC interaction) can only be estimated from part of the available data.
An alternative to performing a complete factorial using blocks is to use a fractional replicate, also called a fractional factorial design. As an example, a half-replicate with four factors would consist of eight rather than sixteen treatment combinations; these might be as follows:
Since only a fraction of the full number of treatment combinations is tested, only a fraction of the full number of main effects and interactions can be estimated. In effect, what happens is that main effects and interactions are confounded with one another. Two such confounded effects are said to be aliases of each other. In the example no main effects are aliases of each other; the main effect of A, for example, is aliased with the BCD interaction. Fractional replicates are used in the exploratory stages of investigations with factors that are continuous variables, where the aim is to find the global maximum (or minimum) of a response surface.
If it can be assumed that there are no interactions then a Plackett and Burman design is appropriate. This design, introduced in a 1946 paper by Plackett and Burman, is very economical in terms of the number of observations that need to be performed. For example, with seven factors (A–G) each having two levels, the seven main effects can be estimated with the following set of treatment combinations:
A design that has more factors than observations may be described as a supersaturated design.